Nathaniel Santiago
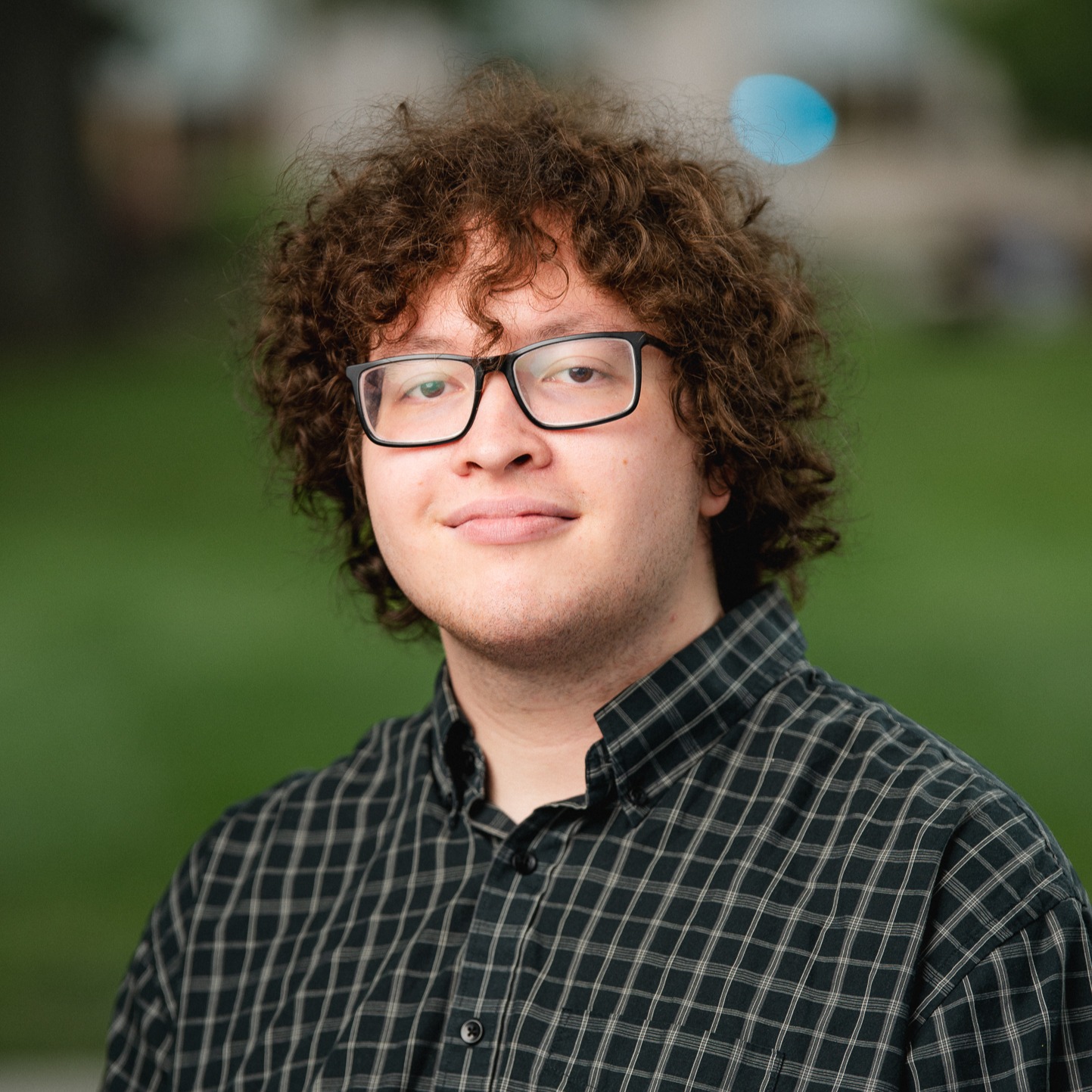
MIT Department: Physics
Faculty Mentor: Prof. Jesse Thaler
Undergraduate Institution: Northeastern Illinois University
Hometown: Chicago, Illinois
Website: LinkedIn
Biography
Nathaniel Santiago is currently a junior studying Physics, Computer Science, and Mathematics at Northeastern Illinois University. He is a McNair, MARC, and HSF scholar. Nathaniel serves as the president of the Society of Physics Students and tutors mathematics at his home institution. In the past, he has collaborated with Dr. Joseph Hibdon to mathematically model and simulate myxobacterial growth phenomena. Additionally, Nathaniel has conducted research in computational particle physics with Dr. Kevin McFarland at the University of Rochester, working on models of neutrino nucleon interactions for the MINERvA collaboration. He is passionate about all topics related to particles, and aims to pursue a PhD in particle physics after completing his undergraduate degree. In his free time, Nathaniel enjoys forcing his friends to watch A24 movies, playing overly complicated strategy games, and suffering as a fan of the Chicago Bears.
Abstract
Graph Neural Network Particle Reconstruction for DUNE’s Prototype
Near Detector
Nathaniel Santiago1,3, Jessie Micallef2,3, Jesse Thaler2,3
1Department of Physics, Northeastern Illinois University
2Department of Physics, Massachusetts Institute of Technology
3The NSF Institute for Artificial Intelligence and Fundamental Interactions
DUNE (Deep Underground Neutrino Experiment) is the upcoming flagship neutrino accelerator experiment in the U.S. that will utilize a novel near-detector design. This design is currently being tested in a 2×2 module arrangement at Fermilab. In order to observe particles that deposit energy outside of the limited 2×2 prototype volume, the detector is being supplemented with 2 collections of scintillator planes, which are detectors from DUNE’s predecessor experiment, MINERvA. The goal of this project is to extend the machine learning architecture that already exists for the 2×2 prototype in order to analyze and incorporate data from the MINERvA components. The training data for this project originates from simulated particle interactions, which is then fed into a detector response simulation for the MINERvA detector components. The output of these simulations is then interpreted as geometric information about particles present in the detector, which is then fed into a graph neural network that identifies particle tracks. This work builds key connections in the framework needed to interpret and understand the results of DUNE.